Business Analytics | Classifier Model and Return of Investment Case
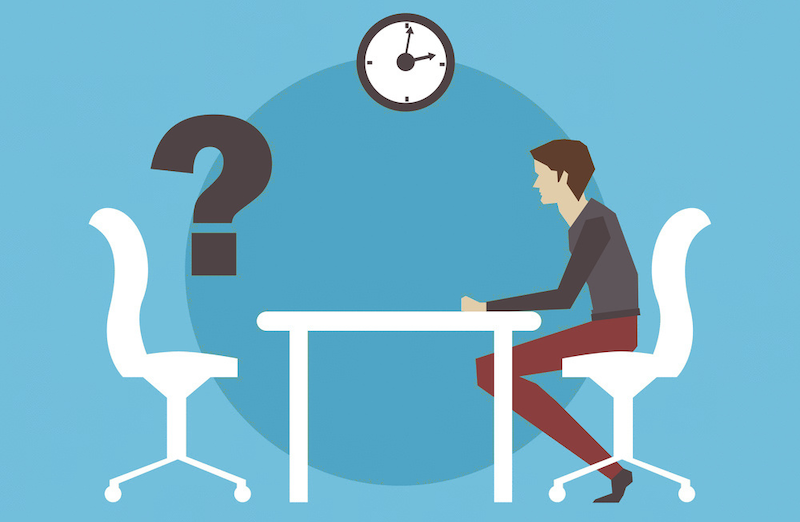
Can you imagine a real business case where you apply machine learning to build and Random Forest classifier and its accuracy of the model isn’t the (only) main metric to pay attention? In real case scenarios the cost and benefits can affect a model in different aspects, this post exercises a business case where the return of an investment is dependent of the behavior of the precision metric.
Intro
Missed appointments cost the US healthcare system over $150 billion a year. Missed appointments directly cause loss of revenue and under-utilization of precious medical resources. It also leads to long patient waiting times and in the long run, leads to higher medical costs.
In this context lets build a predictive model to estimate if an patient will miss an appointment and use the prediction to take an action to try to avoid the cancellation.
Dataset
We obtained a data set with 7,463 medical appointments over a three year period at a specialized care clinic. In this data set, each row corresponds to an appointment and indicates whether it was cancelled or not.
|
|
|
|
Data Overview
Lets see the overall aspect of the data set
|
|
Table: Table 1: Data summary
Name | appdata |
Number of rows | 7463 |
Number of columns | 12 |
_______________________ | |
Column type frequency: | |
factor | 7 |
numeric | 5 |
________________________ | |
Group variables | None |
Variable type: factor
skim_variable | n_missing | complete_rate | ordered | n_unique | top_counts |
---|---|---|---|---|---|
month | 0 | 1 | FALSE | 12 | Aug: 815, May: 791, Jun: 721, Mar: 715 |
weekday | 0 | 1 | FALSE | 5 | Fri: 1733, Tue: 1715, Thu: 1640, Wed: 1448 |
gender | 0 | 1 | FALSE | 2 | F: 5176, M: 2287 |
marital_status | 0 | 1 | FALSE | 4 | MAR: 2504, SIN: 2434, OTH: 1505, DIV: 1020 |
employment | 0 | 1 | FALSE | 4 | UNE: 3824, RET: 1692, FUL: 1370, OTH: 577 |
insurance | 0 | 1 | FALSE | 4 | OTH: 2453, MED: 2366, HMO: 1982, PPO: 662 |
status | 0 | 1 | FALSE | 2 | Arr: 5801, Can: 1662 |
Variable type: numeric
skim_variable | n_missing | complete_rate | mean | sd | p0 | p25 | p50 | p75 | p100 | hist |
---|---|---|---|---|---|---|---|---|---|---|
date_id | 0 | 1 | 525.13 | 303.16 | 1 | 267 | 505 | 791 | 1081 | ▇▇▇▆▆ |
lag | 0 | 1 | 27.24 | 24.47 | 0 | 13 | 20 | 30 | 126 | ▇▂▁▁▁ |
mrn | 0 | 1 | 34251294.01 | 12244201.39 | 7967755 | 28165486 | 41065688 | 42621914 | 44150534 | ▂▁▁▁▇ |
age | 0 | 1 | 54.09 | 18.52 | 6 | 47 | 57 | 67 | 90 | ▂▂▆▇▂ |
time_since_reg | 0 | 1 | 4754.47 | 2399.85 | 179 | 4374 | 5686 | 6196 | 8421 | ▂▁▁▇▁ |
In total, 1662 out of 7463 appointments were cancelled, we can see it from column status (our target).
Model
Since we are interested in appointment cancellations, the target variable is whether an appointment is cancelled or not and success in this particular context means that an appointment is cancelled.
|
|
We build a simple and direct random forest model using Ranger implementation through Tidymodels package.
|
|
|
|
Evaluation
How good is our prediction model?
|
|
.metric | .estimate |
---|---|
accuracy | 0.7599143 |
kap | 0.1613651 |
sens | 0.9216366 |
spec | 0.2099057 |
ppv | 0.7986779 |
npv | 0.4405941 |
mcc | 0.1774102 |
j_index | 0.1315423 |
bal_accuracy | 0.5657711 |
detection_prevalence | 0.8917471 |
precision | 0.7986779 |
recall | 0.9216366 |
f_meas | 0.8557630 |
|
|
Taking a Business Decision to action
With the prediction in hands, we can make a business action to try revert appointments cancellations. Let’s assume we can make a phone call to anyone predicted as “Cancelled” one or two days early and this will revert around 30% of the cancelled appointments. Is it a viable approach? Is it economically viable? At least, better than let the patient cancel his appointment?
To decide this we need to characterize the value of some variables, for this exercise we can assume:
- Cost of a phone call: $5
- Revert appointment cancellation rate: 30%
- Benefit of an appointment: $60
So, we can calculate the Return of Investment (RoI) of this action:
|
|
So we come out with this result:
- Total Cost: 202 * $5 = $1010
- Total Benefit: 89 * 0.3 * $60 = $1602
- Return: $1602 - $1010 = $592
As we saw, with a RoI of $592 this action worth to be take.
One aspect essential to pay attention, we call to all patient predict as “cancellation” but we only get return over 30% (reversion rate) of those truly identified as “cancellation”, a.k.a, True Positives. In other words, the cost of the action is function of True Positives plus False Positives and the benefit of the action is function only of True Positives. This is because a False Positive is a patient predicted as “cancellation” but he´ll go to the appointment, so the phone call does not bring any benefit, only cost in those cases.
Improving Business Performance
Can we do better return without improving the model? As the RoI is function of the rate between True Positives and True Positives + False Positives (a.k.a Precision Metric) in proportion of the benefits and cost we can tune our classifier changing the logistic threshold to change the precision towards to try maximize the RoI.
To see this in practice, calculating what happens with our RoI changing the classifier threshold from 0 to 1 in increments of 0.01:
|
|
threshold | TP | FP | cost | benefit | roi |
---|---|---|---|---|---|
0.00 | 424 | 1442 | 9330 | 7632 | -1698 |
0.01 | 422 | 1098 | 7600 | 7596 | -4 |
0.02 | 408 | 908 | 6580 | 7344 | 764 |
0.03 | 404 | 861 | 6325 | 7272 | 947 |
0.04 | 402 | 843 | 6225 | 7236 | 1011 |
0.05 | 401 | 829 | 6150 | 7218 | 1068 |
0.06 | 401 | 825 | 6130 | 7218 | 1088 |
0.07 | 400 | 818 | 6090 | 7200 | 1110 |
0.08 | 398 | 812 | 6050 | 7164 | 1114 |
0.09 | 393 | 797 | 5950 | 7074 | 1124 |
|
|
We can see the the RoI is better using a threshold between 0.2 and 0.3, and not 0.5 as usually set, more than that, at this level we get worst accuracy for the model, take a look:
|
|
.metric | .estimate |
---|---|
accuracy | 0.6918542 |
kap | 0.2451063 |
sens | 0.7330097 |
spec | 0.5518868 |
ppv | 0.8476343 |
npv | 0.3780291 |
mcc | 0.2535561 |
j_index | 0.2848965 |
bal_accuracy | 0.6424483 |
detection_prevalence | 0.6682744 |
precision | 0.8476343 |
recall | 0.7330097 |
f_meas | 0.7861659 |
Compare this the accuracy metric (0.6918542) with the value obtained in the first calculation above (0.7599143).
Conclusion and Classification Metrics
As we saw, a business decision is not only function of the accuracy of the model, costs and benefits can affect different aspects of the model. Remember to return to real life when applying the model in business case scenarios, to optimize the correct target.
And, be aware of the classification metrics.
|
|